Professor Paula Carney
Textbook: D’Agostino, R.B., Sullivan, L.M., and Beiser, A.S. (2006) Introductory Applied Biostatistics.Belmont, CA: Brooks/Cole, Cengage Learning.
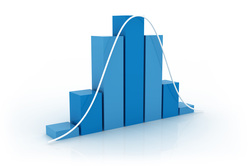
Like most practicing physicians, I regularly read clinical research studies that depend on statistics to analyze their data and draw conclusions. And like most of my colleagues, I probably don’t pay as much attention to the statistical methods and analysis sections as I should. That said, I still have a
reasonable level of comfort with many of the commonly used techniques, and I looked forward to this course as a chance to relearn the details, principles and even some of the mathematics of the statistical methods which are vital to both medical informatics and biomedical research in general. Although some of the students in the class seemed intimidated by the subject matter, Dr. Carney
served us all well by dividing the material into smaller, more easily comprehensible sections arranged on an operational framework which focused on biomedical applications and the interpretation of data. There was just enough theory and mathematics to make it interesting, and it didn’t hurt that the lead author of the textbook was the senior statistical advisor of the legendary Framingham study.
reasonable level of comfort with many of the commonly used techniques, and I looked forward to this course as a chance to relearn the details, principles and even some of the mathematics of the statistical methods which are vital to both medical informatics and biomedical research in general. Although some of the students in the class seemed intimidated by the subject matter, Dr. Carney
served us all well by dividing the material into smaller, more easily comprehensible sections arranged on an operational framework which focused on biomedical applications and the interpretation of data. There was just enough theory and mathematics to make it interesting, and it didn’t hurt that the lead author of the textbook was the senior statistical advisor of the legendary Framingham study.
We began by learning about types of variables (nominal, ordinal, interval, ratio), sampling methods, samples vs. populations, and statistics vs.
parameters. We progressed to descriptive statistics, data distributions, and determining the correct test to analyze particular types of data. We then studied probability, binomial distributions, the central limit theorem, and normal distributions. We next tackled t-tests and the five steps of hypothesis testing which we would apply to every problem set and exam from that point forward:
1. Explicitly state the null and alternative hypotheses derived from the scenario or research problem.
2. Select the appropriate test statistic and state the reasons for choosing that procedure and any assumptions made in applying it to the
particular problem.
3. State the decision rule. What value of the test statistic will allow the null hypothesis to be rejected?
4. Calculate the value of the test statistic.
5. Interpret the result. Was the null hypothesis rejected, and, if so, at what level of significance? What does rejecting (or failing to reject)
the null hypothesis mean in the context of the original problem?
Building on this foundation, the course went on to cover one and two tailed t-tests, chi squared testing, statistical power, type 1 and type 2 errors, ANOVA, dependent and independent variables, correlation and regression, parametric vs. nonparametric tests, making predictions from linear models, and many other topics.
parameters. We progressed to descriptive statistics, data distributions, and determining the correct test to analyze particular types of data. We then studied probability, binomial distributions, the central limit theorem, and normal distributions. We next tackled t-tests and the five steps of hypothesis testing which we would apply to every problem set and exam from that point forward:
1. Explicitly state the null and alternative hypotheses derived from the scenario or research problem.
2. Select the appropriate test statistic and state the reasons for choosing that procedure and any assumptions made in applying it to the
particular problem.
3. State the decision rule. What value of the test statistic will allow the null hypothesis to be rejected?
4. Calculate the value of the test statistic.
5. Interpret the result. Was the null hypothesis rejected, and, if so, at what level of significance? What does rejecting (or failing to reject)
the null hypothesis mean in the context of the original problem?
Building on this foundation, the course went on to cover one and two tailed t-tests, chi squared testing, statistical power, type 1 and type 2 errors, ANOVA, dependent and independent variables, correlation and regression, parametric vs. nonparametric tests, making predictions from linear models, and many other topics.
The course also taught us to use the SPSS software package to do our calculations, freeing us from much of the drudgery I encountered using
statistics in college. With the concepts we learned and the computer to do the arithmetic, even the midterm and final exams were fun (see attached links). Our one project in the course was to write a brief individual research proposal where each student selected a problem in medical informatics, developed a research design, defined the problem's variables and stated hypotheses that could be tested statistically. We then identified the statistical measures used to test the hypotheses, explained why those particular methods were chosen, addressed the issue of checking that any assumptions for the tests were valid, and described how we would interpret the results. A link to my research proposal is
also attached.
statistics in college. With the concepts we learned and the computer to do the arithmetic, even the midterm and final exams were fun (see attached links). Our one project in the course was to write a brief individual research proposal where each student selected a problem in medical informatics, developed a research design, defined the problem's variables and stated hypotheses that could be tested statistically. We then identified the statistical measures used to test the hypotheses, explained why those particular methods were chosen, addressed the issue of checking that any assumptions for the tests were valid, and described how we would interpret the results. A link to my research proposal is
also attached.